An intensive ground survey alone can't keep pace with the rate of change of land use and consequently habitat change over a large area. Remote Sensing and Geographical Information System (GIS) technologies are used to collect information related to the physical parameters of wildlife habitat and geospatial modeling used to evaluate wildlife habitat. The availability of better spatial data has ease habitat evaluation and management more realistic and more scientific. Proper management and conservation of wildlife populations and their habitat depends on the ability to understand the interrelation between the species and habitat interaction. This could be understood with the proper application to assess habitat suitability considering factors influencing the species distribution in the given area.
GIS layers of habitat parameters- continuous distance from grasslands, waterbody, sandbank/ barren land, agriculture/settlement, and categorical land use/land cover map are used as predictor variables. Animal presence location is used as the dependent variable. Based on remote sensed factors, habitat factors, and species presence location habitat is classified as suitable for given species. The habitat suitability map can be prepared based on the study to delineate the area that required specific planning and management intervention. The result obtained from analyzing various factors can be effectively used to enhance suitability in favor of respective species and the overall socio-economic and ecological sustainability that the conservation sector aims to provide.
Why Habitat Suitability Assessment?
Biodiversity is the variability among living organisms from all sources including terrestrial, marine, and other aquatic ecosystems, and the ecological complexes of which they are a part; this includes diversity within species, between species, and of ecosystems (CBD, 1992). It is the foundation of life on Earth as it is crucial for the functioning of ecosystems that provide us with products and services without which we couldn’t live (IUCN, 2012). However, today, every country all over the world has to deal with both biodiversity loss and degradation. Globally, species extinction risk has worsened by about 10 percent over the last three decades, with the Red List Index declining from 0.82 in 1990 to 0.75 in 2015, and to 0.73 in 2020 (a value of 1 indicates no species are at risk of extinction in the immediate future, while a value of 0 indicates all species are extinct). This shows that more than 31,000 species are threatened with extinction due primarily to habitat loss from unsustainable agriculture, deforestation, unsustainable harvest and trade, and invasive alien species (SDG report, 2020).
Until recently many conventional techniques have been applied for collecting data on natural resources. A relatively large number of ground-based studies have been carried out on habitat and corridor use by wild animals. Ground survey methods such as counting animals, trapping, collection of droppings, investigations of feeding sites as well as ground mapping of habitats (Giles 1978; Kotwal & Parihar 1988; Lamprey 1963) will always be useful. However, in a number of cases, remote sensing can supplement or partially replace tedious ground survey methods. Moreover, ground methods have limitations as the whole area can’t be accessed in one go in many of the cases and the information collected may not be as accurate as is possible through remote sensing aided by limited ground survey. In addition, these ground surveys cannot keep pace with the rate of land-use change and consequently habitat composition over large areas. Remote sensing not only provides spatial data but also allows us to compare temporal variations in the spatial data, essential for wildlife management. The application is gaining interest by researchers with various purposes in order to:
- model structural and compositional attributes of various habitat types- link spatial analysis and ecological theory,
- accurately assess large areas of habitat in the management of wildlife,
- allow for rapid qualitative and quantitative spatial assessments in a cost-effective way,
- obtain the opportunity for large-scale modeling to examine the effects of proposed habitats at local and regional scales,
- generate multivariate maps that could be analyzed in a model, and
- quantify multi-dimensional habitat relationships across broad geographical areas
Hence, the quickest possible way for inventory and evaluation of natural resources is through applications of remote sensing and GIS technologies. Remotely sensed data provide capabilities for synoptic, frequent, real-time, and accurate assessment, monitoring, and management of natural resources. With the advancement in the ecological and technological fields, management of natural resources and wildlife has become more scientific and realistic.
Geospatial Approach for Habitat Suitability Assessment
Wildlife managers now have access to the highly accurate spatial database, which enables them to look at the species-habitat relationships in a much better way. With the rise of new powerful statistical techniques and GIS tools, the development of predictive habitat suitability models has rapidly increased in ecology (Guisan & Zimmermann, 2000). Such models are static and probabilistic in nature since they statistically relate the geographical distribution of species or communities to their present environment. Its main purpose is to define the relationship between biotic and abiotic factors and the species spatial distribution (Guisan et al., 2000). It helps to predict the range of habitat variability that will sustain a particular species and through that prediction the potential impact of habitat alteration (Turner et al., 1995). It can also help with describing species-environment relationships as well as derive a map of habitat quality. Further, prediction and mapping of potentially suitable habitats for threatened and endangered species are critical for monitoring and restoration of their declining native populations in their natural habitat, artificial introductions, or selecting conservation sites, and conservation and management of their native habitat (Kumar & Stohlgren, 2009).
There are different approaches to Habitat Suitability Modeling which include Generalized Linear Modeling (GLM), General Additive Model (GAM), Ecological Niche Factor Analysis (ENFA), and Multi-Criteria Evaluation (MCE). ENFA and GLM are examples of empirical models but MCE is a theoretical model (Hughes, 2009). The choice of the most appropriate model depends primarily on the type of response variable (Hirzel & Guisan, 2002). ENFA has been widely used in HS models of presence-only data (Hirzel & Guisan, 2002; Mertzanis et al., 2008; Huck et al., 2010). Both methods (GLM and ENFA) are quite robust and produce equivalent results when the quality and quantity of the data are good (Hirzel et al., 2001).
Problem statement on Geospatial Approach to Habitat Suitability Assessment
The survival of wildlife species depends on the quality of habitat that they use for obtaining food, cover, water, and space. So, for the conservation of wildlife populations, effective conservation and management of their habitats are necessary. The basic objective of wildlife management is to maintain habitats for species as they exist in undisturbed ecosystems or provide habitats where they have been depleted. However, human interference has resulted in a drastic decline in population size and distribution range of many species of wildlife as well as habitat conversion or fragmentation. For example, Himalayan Thar (Hemitragus jemlahicus), which was once common in the mid-hills of Nepal, is now extinct there because of habitat loss and hunting (Green 1979), and Himalayan serow (Capricornis thar) is confined to remote forest areas on the slopes of mountains (Paudel and Kindlmann 2012).
Habitat suitability assessment can be used as prediction and mapping of potentially suitable habitat for threatened and endangered species helps in monitoring and restoration of their declining native populations in their natural habitat, artificial introductions, or selecting conservation sites, and conservation and management of their native habitat (Kumar & Stohlgren, 2009). In Nepal, the tiger population is currently increasing, with numbers having gone up from 98-123 (1999/2000) to 235 (2017/2018). This number has soared in the last four years, as indicated by the 2017/2018 tiger count. As the tiger population continues to rise, habitats become narrower, and the need for immediate expansion and management of future habitats seems apparent. Similarly, with the aim of establishing a second ecologically viable sub-population of Wild water buffalo, the Government of Nepal (GoN) relocated 20 wild water buffalo (5 males and 15 females) to Chitwan National Park (CNP). This strategy, at its very best, can establish another viable sub-population and at its worst, can lead to ecological extinction if any of the catastrophic events occur. Thus, the detailed study of habitat suitability analysis and other conservation issues are important for the conservation of reintroduced wild water buffalo. So, biodiversity and species habitats go hand in hand and effective conservation of wild species populations requires complete knowledge of the habitat requirement of each species as well as an understanding of the relationship between populations and their habitats (species-habitat interactions). Hence, habitat-suitability modeling is being increasingly used as a tool for conservation biology.
The method used for Habitat Suitability Assessment of Large Mammals
The important key for any habitat suitability model is the nature of the species data i.e., presence data, presence and absence data, and abundance data (Eastman, 2006). Figure 1 shows the habitat suitability modeling process. The independent data are the eco-geographical variables (EGVs) of soil, disturbances, and the potential of isolation whereas the species presence or presence-absence data form the response variable. All these variables constitute an input to the statistical habitat suitability model. The two major aims of a Habitat Suitability Model are explanation (“habitat factors ranking”) and prognosis (habitat suitability map). For the explanation part, the habitat factors are ranked by their relevance for focal species habitat choice. The prognosis part provides an area-30 wide estimation of habitat suitability which is the same as the probability of occurrence. The final step of habitat modeling is to do an evaluation to check the quality of model prediction.
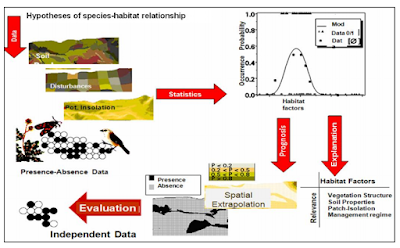
Figure 1: Habitat suitability modeling process (Modified from Schröder and Reineking, 2004).
Habitat Evaluation using GIS and species presence Data
Habitat evaluation constitutes an important and fundamental step in the management of wildlife populations and conservation policy planning. Geographic information system (GIS) and species presence data provide the means by which such evaluation can be done. Maximum Entropy (MaxEnt)is widely used in habitat suitability modeling due to its power of accuracy and additional descriptive properties. Such evaluation constitutes one of the first steps in wildlife protection and management, offering a scientific rationale for the improvement of conservation policies.
In this section we are going to present a case study from (Sharma et al. 2020):
Suitable habitat of the Wild Asian elephant in Western Terai of Nepal
The study was conducted in the Banke, Bardia, Kailali, and Kanchapur districts of western Nepal, with a total area of 9,207 km2. Habitat loss, conflict with humans, electrocution, and poaching were identified as the most prominent threats to elephants. We found out that this study explored how these threats are likely to impact current populations of elephants and the extent and connectivity of suitable habitats both inside and outside protected areas. Research into these factors was deemed crucial to ensure the continued survival of species within the country. The study aimed to identify the important habitat parameters and environmental variables within topographic, vegetation-related, and anthropogenic categories that determine suitable wild Asian elephant habitats in the Western Terai region of Nepal.
For the study, occurrence points of wild Asian elephants have collected then environment, vegetation, and topographic variables were downloaded from various websites. The Topographic included slope, aspect, and elevation. Vegetation-related variables included min, mean and max evi alongside forest cover. Anthropogenic variables included distance to settlement, distance to road, distance to the path, and land use land cover. Accuracies of the model were accessed by two methods: threshold independent and threshold dependent. In the threshold independent method, the value of accuracy was directly obtained from the model, but in the threshold dependent method, the study provided the threshold to maximize the sum of specificity and sensitivity.
The study identified a total of 3,194.82 km2 as suitable habitat for wild Asian elephants in the study area (Figure 2). The study also found that about 39.11% (1,249.58 km2) of this habitat occurs in existing protected areas (Table 1). The largest area of suitable habitat outside protected areas was found in Kailali district (942.55 km2), following Banke, Bardia, and Kanchanpur districts containing 719.46 km2, 798.67 km2, and 734.14 km2, respectively. Bardia National Park and its Buffer Zone were found to contain the largest proportion of suitable habitat (46.84%), with Krishnasar Conservation Area containing the smallest portion (0.16%). Elephant habitat in the study area was found to be highly fragmented, occurring as small, discrete patches. Connectivity between habitat patches was found to be low in the southern and northern parts of the study area, but higher in the center. The most influential variables for habitat suitability were the distance to road, distance to water, elevation, and slope. Distance to settlement, and mean EVI and LULC were identified as the least important variables. The model, therefore, indicates that elephants prefer habitat far from roads, near to water sources, with low elevation and gentle slope.

Figure 2: Predicted suitable habitat of wild Asian elephant based on MaxEnt modeling.
(a) Banke National Park and its Buffer Zone
(b) Krishnasar Conservation Area
(c) Bardia National Park and its Buffer Zone
(d) Shuklaphanta National Park and its Buffer Zone
Table 1: Suitable habitat for wild Asian Elephant in protected areas
Protected area | Total area(km2) | Elephant's habitat (km2) |
Shuklapanta National Park and its Buffer Zone | 548.5 | 352.09 |
Bardia National Park and its Buffer Zone | 1,475 | 585.28 |
Banke National Park and its Buffer Zone | 893 | 310.22 |
Krishnasar Conservation Area | 16.95 | 1.99 |
Total | 2,933.45 | 1,249.58 |
Therefore, this study identified the suitable habitat for Asian elephants and acknowledged the importance of the most prominent variables for the habitat suitability of this species.
Conclusion
Habitat suitability assessment using geospatial approaches is not a perfect representation of large mammal distributions. Yet, in the present context, the availability of point occurrence data is very limited, and this is unlikely to change substantially any time soon. Geographical ranges based solely on expert knowledge are highly subjective and their level of detail is variable according to the geographical region where a species lives, the size of its range, and its primary habitat. Habitat suitability models improve on geographical ranges because they make inference explicit, easy to evaluate quantitatively, and more uniform across species, thereby reducing bias in mapping species distribution. Furthermore, habitat suitability models also can track changes in the extent of suitable habitat over time as maps of land-cover change are updated and can be used to make explicit predictions on future habitat loss, which can make conservation assessments proactive and more effective in preventing future threats. Habitat suitability models greatly enhance the resolution of mammal distribution analysis and provide important baseline information for landscape-level conservation over a broad altitudinal range. Also, these habitat suitability mapping approaches can provide necessary information for designing regional sustainable management and conservation planning, as well as evaluating the existing system of protected areas in the study region.
References
Eastman, J. R. (2006). IDRISI Andes guide to GIS and image processing. Clark University, Worcester, 328.
Giles Jr, R. H. (1978). Wildlife management. Wildlife management.
Green, M. J. (1979). Tahr in a Nepal national park. Oryx, 15(2), 140-144.
Guisan, A., & Zimmermann, N. E. (2000). Predictive habitat distribution models in ecology. Ecological modelling, 135(2-3), 147-186.
Hirzel, A. H., Helfer, V., & Metral, F. (2001). Assessing habitat-suitability models with a virtual species. Ecological modelling, 145(2-3), 111-121.
Hirzel, A., & Guisan, A. (2002). Which is the optimal sampling strategy for habitat suitability modelling. Ecological modelling, 157(2-3), 331-341.
Huck, M., Jędrzejewski, W., Borowik, T., Miłosz-Cielma, M., Schmidt, K., Jędrzejewska, B., ... & Mysłajek, R. W. (2010). Habitat suitability, corridors and dispersal barriers for large carnivores in Poland. Acta Theriologica, 55(2), 177-192.
Huges, A. 2009. Expert Driven Habitat Suitability Modeling for the Magellanic Woodpecker in Karukinka National Park Tierra del Fuegl, Chile. M.Sc thesis: pp. 1-59.
IUCN. 2012. Available from: http://www.iucn.org/what/tpas/biodiversity/about/.
Kotwal, P.C. & J.S. Parihar. 1988. Management Plan of Kanha National Park and Project Tiger, Kanha for the period 1989-90 to 1998-99. Report, Mandla.
Kumar, S., & Stohlgren, T. J. (2009). Maxent modeling for predicting suitable habitat for threatened and endangered tree Canacomyrica monticola in New Caledonia. Journal of Ecology and the Natural Environment, 1(4), 094-098.
Lamprey, H.F. 1963. Ecological separation of large mammal species in the Tarangire Game Reserve, Tanganyika. East African Wildlife Journal 5: 151- 166.
Mertzanis, G., Kallimanis, A. S., Kanellopoulos, N., Sgardelis, S. P., Tragos, A., & Aravidis, I. (2008). Brown bear (Ursus arctos L.) habitat use patterns in two regions of northern Pindos, Greece–management implications. Journal of Natural History, 42(5-8), 301-315.
Paudel, P. K., & Kindlmann, P. (2012). Human disturbance is a major determinant of wildlife distribution in Himalayan midhill landscapes of Nepal. Animal Conservation, 15(3), 283-293.
Sharma, P., Panthi, S., Yadav, S. K., Bhatta, M., Karki, A., Duncan, T., ... & Acharya, K. P. (2020). Suitable habitat of wild Asian elephant in Western Terai of Nepal. Ecology and Evolution, 10(12), 6112-6119.
Turner, M. G., Arthaud, G. J., Engstrom, R. T., Hejl, S. J., Liu, J., Loeb, S., & McKelvey, K. (1995). Usefulness of spatially explicit population models in land management. Ecological Applications, 5(1), 12-16.
-- A group work of Binaya Adhikari, Namrata Devi Khatri, Sandaya Kandel, and Dipendra Kshetri was submitted to the university as an assignment.
0 Comments